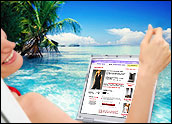
More is better, right? Wrong. In The Paradox of Choice: Why More is Less, Barry Schwartz explains how too many options actually cause more psychological distress than good. And nowhere is the overabundance of choice more prevalent than the Internet, where any given Web site can present us with an overwhelming number of alternatives at once.
The solution is not carrying fewer products or content. One of the beauties of the online world is that there’s something for everyone. As Chris Anderson explains in his popular book, “You can find everything out here in the Long Tail.” It is within this plethora of options where the content and products that truly meet people’s needs are found, and where companies ultimately make more money.
So if carrying less is not the answer, what is? The online world has devised numerous strategies in an attempt to guide users to products and content that will best meet their needs. Many large sites employ the efforts of skilled merchandisers or editors armed with aggregated analytics data to help point the way, and others rely on crowdsourcing techniques such as ratings and reviews to narrow down the choices.
While the above methods can be valuable in navigating the quagmire of choice, they all suffer from one major problem: bias. Bias comes in a number of guises, and we will walk through seven of the most common and detrimental here. In the end there is hope, though, as there are new technologies capable of largely evading these biases.
Personal Bias
The human brain fundamentally approaches the world in a self-centered way; we see the world through the filter of our past experiences and knowledge as well as our own interests and attitudes. But since we do not have access to all of the information that drives another person’s attitudes and behaviors, we are often wrong when we attempt to predict what another person or group of people will find interest in.
One way to mitigate the problem is to run a focus group. Though this can help remove the biases of the expert, members of a focus group suffer from personal bias as well, such as when a dominant opinion influences the entire group. Additionally, you will never have a truly representative sample of people and so will be swayed by the luck of the draw on the attitudes of the specific people you have chosen. The bottom line is, whenever you use a small sample of the population — be it an expert or a focus group — to predict the greater population, you need to recognize the influence of personal bias.
Squeaky Wheel Bias
Crowd-sourcing, such as ratings and reviews, has become a popular technique for creating recommendations online. In theory this approach has few flaws: If every single person who came to the site weighed in with their opinion on every product, you would get a perfect representation of consumer attitudes.
The problem, of course, is that not everyone contributes. The tendency is for certain kinds of people to make their voice heard, particularly when effort is involved (such as in a review).
The most vocal and misleading group of contributors is what I like to call the “squeaky wheels”. This could be those people who simply like to complain. But it can also be any one of us when we have a negative experience. Negative experiences tend to stand out more than positive ones and also encourage us to take action.
Overly positive reviews happen too, but again what happens is that you get a representation of the community that is biased to the two extremes. Five scathing reviews, three glowing ones, a few people who just like to be heard. At the end of the day, 99 percent of the population remains unspoken for.
Contextual Bias
Another challenge with relying on explicit user feedback is contextual bias. Imagine, for example, reviews of a digital camera. One person might say that the “resolution is incredible” and another that “resolution sucks.” They may both be right. Perhaps one person uploads their pictures to the Web, where the resolution is great, and another likes to print them out where higher resolution is needed. The two reviewers are using the camera and rating it from two different contexts. Ratings have no way of reflecting such nuances and reviews only do if the person writing it is very aware of their contextual bias.
Emotional Bias
Our emotional state colors our perception and experience of everything we come in contact with, biasing our responses and opinions. And how we feel can change day by day or even minute by minute depending on a variety of external and internal influences. We have all had the experience of disliking a movie when we were in a bad mood only to discover months later that it’s actually quite good.
If we are providing feedback that is simultaneous with our experience of a product or service, our emotional state at the time of evaluation can have a major influence on our evaluation. This is also part of the reason why asking people what they think is so often a poor predictor of what they actually do.
Gaming Bias
Another type of reviewer is someone who is “gaming” the system. Sometimes such gaming is malicious, but often it’s altruistic. While writing this article I went onto Amazon to look at the reviews of a book I co-authored called Wired for Speech. The first one was very positive; perhaps someone my coauthor knows. But I have no doubts about the second 5-star review, titled “Amazing Insight.” To my surprise, it was from my dad! Enough said.
Gaming such as this is actually the rule, rather than the exception on Amazon and other media sites where products have authors or artists and personal connections abound. I admit to having given 5 stars to articles on my company … heck, if I can do it for this one, I will. Go ahead, try it out, give this five stars if you can!
Time Delay Bias
A problem that layers on top of all the above has to do with time. Unfortunately, feedback is never completely up-to-date. By the time the data is collected and analyzed, the recommendations may be wholly inaccurate. Trends and fads come and go in a matter of weeks or even days. News stories rise and fall in popularity in a matter of hours or minutes; it is nearly impossible to keep up unless you use a technology that automatically adapts recommendations in real-time.
Ratings and reviews can suffer from similar time-delay problems. Imagine a scathing review of a small bed-and-breakfast that the owner has made changes to accommodate and therefore is no longer valid. My wife and I stayed at a bed-and-breakfast in New Zealand where this is precisely what happened. The owner was distraught and felt it had a negative impact on his business even though he had made all the changes he could to accommodate.
Multiplier Bias
What if everyone’s movie decisions relied solely on the basis of how many other people have seen it or how much money it has made? The situation degrades into “herd behavior” and effectively becomes random group movements. This can happen any time the feedback mechanism is tied to the action itself. For example, recommending products based on which are purchased most often. The more who purchase it, the more encouraged to purchase it. Even if mechanisms are in place to take return into account, not everyone returns a bad product. Things become self-fulfilling.
Recommendation systems based solely on clicks and page views have a similar problem. The more who click on a page, the more others are encouraged to do the same. It quickly degrades into randomness.
Tapping the Wisdom of Your Silent Majority
With all of these biases, should we completely avoid strategies like expert recommendations and user-generated reviews? Not necessarily. If done with appropriate care, merchandising and editorializing can be helpful guides. Ratings and reviews, though potentially misleading, have become an expected part of the user experience online and encourage deeper engagement with and consideration of products and services.
There is another strategy, however, which sidesteps bias and provides both an accurate and comprehensive window into user need and interest by leveraging the wealth of information embedded in the everyday online behaviors of Web site visitors. Every successful or failed search, every page visited or revisited, every purchase or abandoned cart, carries with it valuable information that is typically ignored or relegated to reports with unclear consequence. These natural online behaviors represent your true and unbiased community — the “silent majority” of your Web site visitors that normally go unspoken for.
Through analyzing the patterns embedded in these implicit community behaviors, and then automatically modifying both on-page recommendation links as well as search results, companies can effectively tap into this community wisdom. When a user comes to your Web site with a particular need or interest, focus on the experiences of the entire visitor community to identify other like-minded peers and immediately surface the products and content that have proven valuable in the past.
Watch what people do (not what they say), include everyone, and pay particular attention to context. That is what behavioral science has always told us is the best way to understand a community, their needs, and interests. Bias may never be 100 percent avoidable, but by tapping into the wisdom of your silent majority, it is possible to guide visitors to content or products that satisfy their needs much faster than ever before.
Scott Brave, Ph.D is a founder and CTO of Baynote. Prior to Baynote, he was a postdoctoral scholar at Stanford University and served as lab manager for the CHIMe (Communication between Humans and Interactive Media) Lab.
Social Media
See all Social Media